What Biologists can learn from LLMs?
Ashwin Gopinath, Biostate AI, Inc.
(ashwin.gopinath@biostate.ai)
Dec 4, 2023
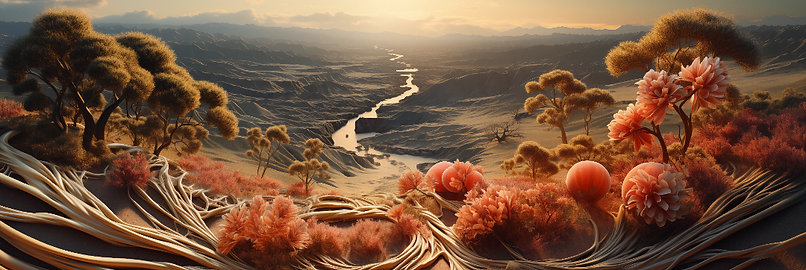
"All models are wrong, but some are useful"
-George E.P. Box
Large language models (LLMs) like ChatGPT, over the last year, have fundamentally changed the way we interact with technology, due to their ability to generate grammatically accurate content in multiple languages from simple prompts. This transformation, appearing almost magical, is the culmination of nearly five decades of evolution in natural language processing (NLP). NLP's journey began in the late 1960s with rule-based systems, constrained by rigid grammar and syntax rules. It transitioned to statistical models in the 80s and 90s, exemplified by consumer-facing technologies like T9 predictive text. The real leap in NLP came with neural networks in the early 2000s, inspired by the human brain, enabling models to learn from vast datasets without explicit rules, thereby enhancing contextual understanding. The introduction of the Transformer architecture in 2016, highlighted in the paper ‘Attention is All You Need,’ marked a pivotal moment. This innovation revolutionized NLP by allowing models to comprehend the relational significance of words and sentences in a larger narrative, leading to the development of advanced LLMs like GPT, trained with minimal supervision on extensive text corpora.
Building on this concept, the transformative advancements in unsupervised model development in NLP offer a blueprint for understanding and interpreting complex systems, akin to those encountered in biological research. Just as transformer-based models have mastered the intricacies of language, their principles can be applied to the vast data being created in biological research, particularly in the wake of the omics revolution. At first glance, human language and biological systems might appear vastly different, yet they share numerous striking parallels, both being dynamic, interconnected networks rather than mere collections of isolated components. In language, words weave together to form structured narratives, while in biology, biomolecules interact within organisms to create the essence of life. The lifecycle of an organism, characterized by the continuous interaction of biomolecules under the influence of external factors, parallels how sentences build upon each other to form coherent narratives.
This analogy underpins systems biology, which, like NLP, is gradually shifting biological studies from rule-based methods to a holistic approach. Traditionally, biological research primarily focused on discrete, isolated observations, delving into the intricacies of single genes or proteins and then exploring how these elements fit into the larger context of an organism's biology. This approach is reminiscent of analyzing language by scrutinizing individual words in isolation, seeking to understand their broader meaning and role within the full narrative. However, just as NLP has evolved to embrace the complexity of language, systems biology is increasingly recognizing that life processes are the product of complex networks of interactions. This holistic perspective acknowledges that biological functions stem from the synergy of multiple components, not solely the properties of individual parts. Looking ahead, we can anticipate a significant increase in the application of generative AI in systems biology. This paradigm shift holds the promise of profoundly enhancing our understanding of biological processes, using models capable of integrating and interpreting extensive, multifaceted, and likely unlabelled biological datasets, a progression mirroring the advancements in NLP.
AI and machine learning, particularly transformer-based approaches, have already started impacting biological research, especially in protein and drug design. Notable examples include DeepMind’s AlphaFold, Meta’s ESMFold, and Generate Biomedicines' Chroma. These tools, leveraging large datasets on protein structures, have achieved remarkable results of enabling denovo protein design, yet they represent only a fraction of the broader biological puzzle. Designing molecules in biology is comparable to crafting the perfect word in a language – both are precise and highly specialized feats. However, this success in molecule design is just one aspect of a larger challenge. Proteins and other biological molecules don't operate in isolation but within intricate systems. Therefore, predicting their efficacy in biological systems remains a complex and daunting task. Recognizing these complexities, and driven by a desire to bridge the gap between theoretical understanding and practical application, led to the inception of an ambitious project.
In response to these challenges, and fueled by the insights gained from AI's success in language models, Dave Zhang and I co-founded Biostate.ai. This venture operates on the premise that biology, inherently generative and evolving, suggests that a living system's current state results from its previous state and interactions with its environment. By accurately recording a system's internal state and relevant environmental information, predicting its next state becomes feasible. This raises two critical questions: (a) What dataset can give a sufficiently detailed description of a biological system's reality? and (b) What model architecture is suitable for predictions? Transformer-based architectures, known for their effectiveness in language tasks, appear promising for biological predictions. The ideal dataset for this endeavor should be high-dimensional, capturing the complexity of living systems, and include genomic, proteomic, transcriptomic, physiological, and environmental data—a combination we term the ‘biostate.’ While advanced technology for data collection exists, the cost remains a barrier for high-frequency data acquisition.
Building upon this foundation at Biostate.ai, we have embarked on a couple of preliminary research initiatives to demonstrate the potential of our approach. The first study is titled ‘High Frequency Longitudinal RNAseq Reveals Temporally Varying Genes and Recovery Trajectories in Rats.’ In this study, we showed that longitudinal RNA-seq performed on rats, dosed with various drug molecules, can be an effective technique to uncover unique insights. We identified over 4,000 genes that temporally changed significantly after the rats were exposed, allowing us to rapidly distinguish between unhealthy rats and those recovering, with great accuracy. Interestingly, we noticed that rats recovering quickly exhibited different gene activity patterns compared to those recovering slowly, indicating how longitudinal analysis of high-dimensional biostate can be instrumental in understanding biological systems. The second project titled ‘Bioformers: A Scalable Framework For Exploring Biostates Using Transformers,’ introduced a framework applying transformer-based unsupervised models, akin to BERT and GPT, to analyze the ‘biostate’ of living systems. We further tested the efficacy of the model on a relatively small dataset of single-cell transcriptomics, demonstrating our model’s ability to glean significant biological insights like gene network inference as well as molecular even from small datasets. The work also sets up a foundation to enable inferences from longitudinal data as well as to enable transfer learning based on data collected from different species.
As we look to the future, our team at Biostate.ai is embracing the wisdom of George E.P. Box's words: “All models are wrong, but some are useful.” In our journey, we are constantly reminded that while our models may not be perfect representations of the vast complexity of biological systems, they hold immense potential for groundbreaking insights. On the experimental front, our primary goal is to reduce the cost of collecting comprehensive ‘biostate,’ recognizing that affordable and extensive data access is crucial for breakthroughs in this field. In parallel, our focus on AI development involves an ambitious plan: over the coming months, we aim to pre-train our models on the vast array of publicly available multi-omic datasets. This initiative is not just about expanding our database; it's a stepping stone towards mastering transfer learning, which will enable our models to adapt across different types of biological samples. Furthermore, we are refining our models to more effectively process and learn from longitudinal time-series data. We believe that these combined efforts will not only transform the way longitudinal data is perceived in biology but also pave the way for a deeper, more synergistic integration of AI and biology. This work is much more than a technical pursuit; it represents a concerted step towards understanding life's complexities while enhancing human health and environment.
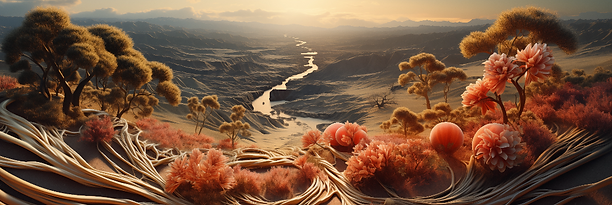