Biology as Clockwork
David Zhang, Biostate AI, Inc.
(dave.zhang@biostate.ai)
Nov 27, 2023
The behavior of a wind-up (clockwork) toy is generally fairly predictable when you put it on a flat terrain and let it go. Maybe it goes straight indefinitely, or maybe it turns about-face after a pre-defined number of steps. But if we put obstacles in its path, then things become chaotic: maybe the toy steps over the sock without noticing, maybe it trips and falls never to get up again, or maybe it staggers before recovering. The outcome depends on the size of the obstacle, the internal mechanics of the toy, and the relative positioning of the obstacle to the toy's gait.
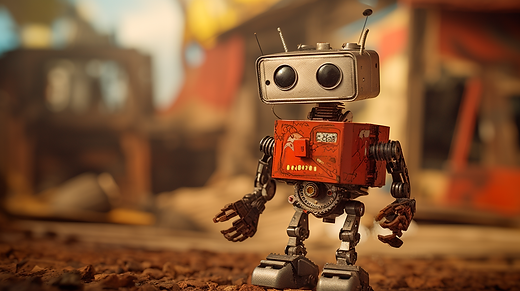
Wind-up Toy, original creation drawn with Midjourney.
It turns out the wind-up toy is a good analogy of an organism's biology -- the clockwork that comprises and moves the toy are akin to the genes and the genes' mRNA and protein products of an individual. In the course of normal everyday living, biology follows a fairly predictable path. Some genes are constantly on like the turning of gears; other genes turn on and off like the rise and fall of the toy's feet. Biology can start to go awry due to perturbations such as exposure to toxins and pathogens, just as the toy's position and orientation is potentially changed by obstacles in its path. A big enough toxin exposure kills the organism, just as a big enough obstacle topples the toy irreversibly. Additionally, the obstacles and perturbations that cause gross disruption can be internal -- such as loose cogs and cancer.
A corollary of the similarity between clockwork and biology, if you accept the premise, is that the future trajectory of the organism or toy are predictable, if you're given a movie of its recent past and its current state. Importantly, predicting the future states does not require you to fully know or understand the internal gears and mechanisms (in the case of the clockwork toy) or the gene regulatory networks (in the case of a living organism). Next token prediction in the absence of full mechanistic interpretability happens to be what modern large language model (LLM) AI excel at: Given an input prompt, the set of tokens provided by the user, the LLM will generate additional tokens one at a time, with each new generated token serving as part of the input for generating the next token. We believe that we can do the same for biology.
To do this, however, we need enough information to analyze and train on. We're not going to have much luck predicting whether the toy topples based only on an instantaneous gyroscope reading. We will need reasonably high-resolution images of the entire toy and the obstacle.
The analogous information required for predicting biology forward is gene expression data. Humans and rats both have about 25,000 genes, and at any given moment for a specific individual, each gene's expression level can be different, varying up to 7 logs. We can almost think of it like a 160 x 160 pixel grayscale image, with each pixel having an intensity property corresponding to the log gene expression level. If we have enough gene expression "pictures" of an individual taken with regular timing, then we may be able to reasonably generate the pictures corresponding to the next few days.
The problem is that this type of data has not really been collected before. Because RNA sequencing (RNAseq) is expensive, researchers typically will only take a picture before the patient or animal model is dosed with a drug, and one more picture after dosing. This is like taking a picture of the clockwork toy before it steps onto the obstacle, and after it either topples or recovers. From this pair of pictures, you can determine whether the obstacle toppled the toy, but you can't figure out how the toy moves, topples, or recovers.
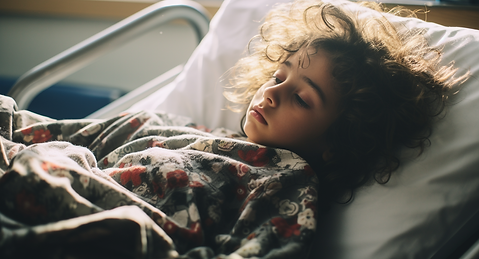
Sick Child, original creation drawn with Midjourney.
That's why we set out to collect our own data. Our first experimental results, now available on biorxiv [1], analyze the daily RNAseq expression profiles of rats before and after dosing them with 4 FDA approved drugs known to cause liver damage at high doses. This was the first experiment of its kind, analyzing 829 blood sample RNAseq data from N=84 rats. Though small in comparison to the scale of our future studies, this is already 4x larger than the previously largest rat RNAseq dataset on the NCBI Gene Expression Omnibus (GEO) database [2].
Preliminary analysis of our data supports the analogy of biology as clockwork. In healthy rats, most genes are expressed stably and a small group of 300 genes appear to naturally vary periodically in expression. When the rats are given small doses of each drug, some genes are up-regulated or down-regulated in response, but these responses last only 2-3 days before the overall gene expression profile returns to normal. As we increase the dose of the drugs given, more and more genes are turned on or turned off and the gene expression profile veers farther and farther away from the healthy region of principal component space.
At high doses of valproate (an epilepsy drug), 2 of the 15 rats in the group died and the other 13 returned to health. The 13 rats that survived could further be subdivided into a group of 4 that recovered quickly and 9 that recovered slowly. This is similar to the wind-up toy encountering an obstacle of intermediate size: depending on the orientation and the position of the obstacle, the toy could step over the obstacle and be completely unaffected, graze it and be briefly disoriented but recover, or trip over it and be permanently toppled. A well-trained generative AI could, in principle, accurately generate the outcome trajectory by analyzing the past motions of the toy and the position/orientation of the obstacle.
At Biostate AI, our mission is to build a generative AI that can analyze past biological state (biostate) information, including RNAseq, to generatively predict future biostates. These future biostates are like a movie of the default outcome, an oracular vision of what will be if nothing changes. If that future is undesirable, such as a patient dying of a disease or suffering from the adverse effects of a new drug, then we can try to change the future by changing the perturbations (drugs and treatments). As humans, we have agency to change the world for the better using tools that we build.
Let's work together to build the AI tool to help everyone live longer and healthier lives.
[1] High Frequency Longitudinal RNAseq Reveals Temporally Varying Genes and Recovery Trajectories in Rats. https://doi.org/10.1101/2023.11.21.568082
[2] NCBI Gene Expression Omnibus (GEO) Database. https://www.ncbi.nlm.nih.gov/bioproject/PRJNA1026523